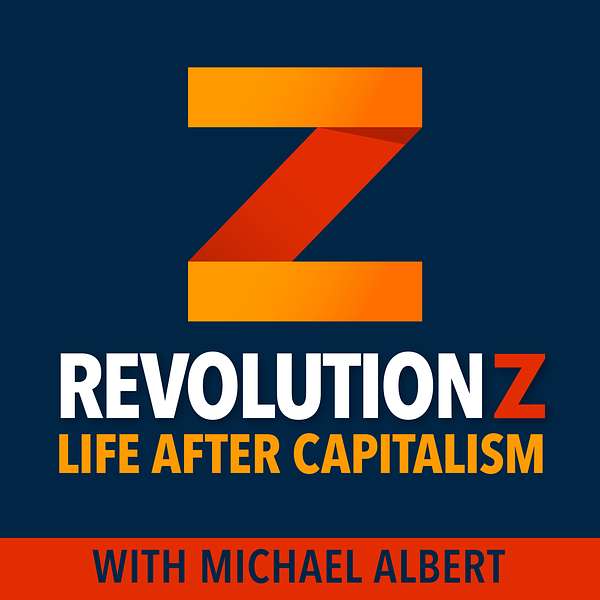
RevolutionZ
RevolutionZ
Ep 294 AI and Society with Evan Henshaw Plath
Ep 294 of RevolutionZ has for a return engagement as guest Evan Henshaw Plath, popularly known as Rabble. We talk about AI and society, first describing where we are now and how we got here, and then likely impacts on various sectors, from software development to music, and video generation. What is the role of training data? How intelligent can AI get? Will it attain AGI? Is there an existential threat? What are the more immediate social and ethical risks and rewards? What are AI researchers like? As a community, do they have cultural connections or even share a quasi religious culture? Do they have in mind more humane and equitable societies? Or is profit in command? Will AI be a tool that enhances life possibilities for people, or will people be infantilized by AI doing too much of what humans ought to do? Can the public impact the outcome?
Hello, my name is Michael Albert and I am the host of the podcast that's titled Revolution Z.
Speaker 1:This is our 294th consecutive episode and also our second of this week, something I only very occasionally do. Our topic this time is AI, artificial intelligence and society, and our guest back for a return engagement on Revolution Z is Evan Henshaw-Plath for return engagement on Revolution Z is Evan Henshaw Plath. Evan, known as Rabble, is a pioneering technologist and activist renowned for their work in social media and decentralized technologies. As the first employee and lead developer at Odeo, where they helped develop Twitter, rabble has been at the forefront of digital communication innovation. Their commitment to user-centric community-driven platforms is evident in their work with Nost Social, which is a decentralized social media app using the Nostr N-O-S-T-R protocol, and their role in relaunching Causescom to empower grassroots activism. They also helped create the Indymediaorg tech team, advancing grassroots media. A former researcher at the MIT Media Lab Center for Civic Media and an Edmund Hillary Fellow, rabo combines technical expertise with a passion for political organizing and social justice, envisioning a future where digital communities thrive independently of centralized control, focusing on building digital commons-based communities. So, evan, welcome back to Revolution Z.
Speaker 2:Thank you so much. I'm happy to be here.
Speaker 1:Quite a while ago, I did a couple of episodes there may even have been a third, I don't remember and one with another guest, arash Kolhai of Znet, the other's just me, about AR. But time has passed and in this domain it's a considerable span of time and it seemed like a good idea to check in on the topic. Indeed, during an earlier session with Evan, I mentioned that possibility of his coming back on to talk about AI. And so here we are. So welcome back again, and to start at first, without assessing or predicting, how would you describe I guess sort of how would you situate the current state of AI? Where is AI at now, roughly? What can it do? What is it doing already? Before we get into more assessment?
Speaker 2:So that's a fascinating question.
Speaker 2:So at the moment there's a tremendous amount of research and investment and projects going forward in AI and it's captured the vast majority of Silicon Valley and startups, investment focus and opportunity and money, and there's tremendous hope that the current set of AI will change huge parts of the economy and society and business.
Speaker 2:And all of these large companies are chasing something they call artificial general intelligence, which is the idea that the AI wouldn't just be about content or creation or be able to occupy specific niches, but would be able to replicate the intelligence of an average human, and the goal behind this movement is that a huge amount of our current economics or current economy could be shifted over to these AIs where you don't have a per hour labor cost, where all of the labor cost is up front, and so we're seeing huge adoption of this stuff in particular areas, and so, and in particular, the more intellectual the labor, the more it gets used.
Speaker 2:So almost every software developer today is using these stuff People who are creating ad copy, people who are creating social media content for websites. It's ubiquitous in that area and we're seeing it in other bits of an area where it is really having a large effect on sort of any kind of piecemeal intellectual work where you know if it can be segmented off and used in a particular segment. It does, and then it becomes massively cheaper to do that work by AI and the people who were paid to do that work lose their jobs.
Speaker 1:That is sort of where we're at. I think it's ubiquitous more than people realize. I think and it's surprisingly because I bet some years ago if you had asked even somebody in the field where do you think AI is going to have its big initial impact, they would have said on rote tasks, on rote labor and even on physical work like warehousing and stuff like that. And it's been the opposite. I've watched videos where somebody is what do you call it prompting?
Speaker 1:Yeah it's prompting the AI to create a video, and the damn thing does it. Or to create a song, and it does it. And and a story, and it does it. So those areas, and clearly programming, are already inundated, and many others are coming. Okay, so what do you expect? Have we hit a plateau? Is it over? Or what do you expect to be the trajectory? Again, without evaluating, just in terms of having a feeling for what's coming, what do you think is coming?
Speaker 2:So at the moment, things are still getting much better, much faster, and there's areas in which, in the current systems, you can tell that it's AI generated, that it's AI generated. There's a particular set of language and words and sort of sentence structure and grammatical forms that the AI engines create that aren't the way humans talk or write, and so there are specific words that you can tell. You know, there's things where you see AI is, in the image generation, really bad at hands and it's bad at continuity between things. You see these AI generated videos of you know people dancing or walking and like the torsos swap or there's a leg that appears, things like that. So at the moment, what happens or what generates text and the text looks great but it's garbled and it's not quite an English word and it's because the translation between these things gets lost and that those things will all be fixed because they're easy for the engineers to see their problems that they could solve, and so the output that we can see is going to get better.
Speaker 2:We run out of training data because the way these things work to learn and build the models is you try and get as much data as you can from humans in there, and my understanding is that the AI companies are reaching the limits of human-created content. You know, they've transcribed. They put all the transcripts of everything on YouTube, every printed book, everything written on the internet. They can find all these private datasets and they're putting them in there and they're training them and that's what they've got right now. And so they claim that they don't care about copyright because they can just throw it all in there and it'll be OK. But what we don't know is can the engineers building the AIs make them better in ways that aren't giving it more data? And so some of these AI companies are like I know, let's just get the AIs to generate more data, to use it as the training set, and that leads to Alice in Wonderland type crazy. The AI is just like going off it hallucinates and then it learns based on its hallucinations, and that's wacky, and we know that might not be a good idea.
Speaker 2:What other folks are doing is saying well, maybe if we had more processor power, maybe if we take more of these GPUs, these sort of neural engines, these special chips, and process it more, we'll be able to do something more interesting to that, and the amount of money being spent on this is huge.
Speaker 2:Nvidia, the company that makes these things, is one of the biggest corporations in the world, and each of these companies are spending many, many billions of dollars on these things. And Sam Altman, the sort of very problematic CEO of OpenAI, talks about needing a trillion dollars to train these things, to train these things. And so if we think on the level of where are we allocating things in the human economy, a trillion dollars is an absolutely massive amount of money. And you would only do it if you really truly believed and could convince people that you're going to have multiple trillions of dollars of return on that investment. And the only way to do that is to take a huge amount of human labor and replace it with labor that can be done by a few corporations that have particular monopolized control over that ability of that labor, and so like they really believe it and they're able to do it and maybe it's true and maybe it isn't, but if it, is true, it has profound implications, right?
Speaker 1:I don't think they would say that, depending upon where they are, they might say instead I think you're right, by the way they might say instead well, we're going to cure cancer, we're going to allow people to do this X, y and Z, and that's the value that the trillion dollars will generate. Yeah, but the reality, I I think, is that you're right. They might say that and they might put that in brochures, but what they're actually looking at is can we translate this entity, this AI thing, into trillions of dollars of profits for a relatively small sector of of companies? So I think you're right about that and the economic side of that. But the other thing that was interesting that you said I just want to go back to before we go forward was the, the inclination of critics, of commentators let's call it, let's not even call it critter you know people trying to evaluate it honestly, trying to evaluate it, to ignore that it's changing. So, in other words, you know you can imagine somebody saying well, you give the example of somebody's leg misplaced, or of you know various other bits of inanity misplaced, or of you know various other bits of inanity. The early one was the New York times guy who the uh, you know the the AI wanted to what was it? Marry him and have him kill his wife or something, that kind of stuff. The AI was saying well then, it's not going to get any place, there's nothing to worry about, it's just stupid. Well, they're stupid, it's the best I can tell. Yeah, because unless, unless there is a, a ceiling, that that is because of physics or because of technology, or because of the amount of space or, as you say, the amount of training data although I don't get that and I want to ask you about that these problems will be overcome.
Speaker 1:Yeah, but the training data. I don't really understand that. It's hard for me to believe. Imagine we could pile all the training data that they have in a pile. Yeah, suppose you took half of it away and you trained it on that. Why would it be any different? In other words, it's mostly redundant and it's. I just don't understand why. Why more and more data? If they discovered a million people tomorrow on an island someplace who've been producing books or or talks, or yeah, and they shove that in, is it really going to be any different?
Speaker 2:It will actually add to it, because, even though these AI engines are designed based on neuroscience research, they work fundamentally differently. They don't work as having this kind of neuroplasticity brain that trains on you know, creates conceptual models and has neural cortexes and that kind of thing, and instead what they work on is this model of you know, repetitive cycles of these neural engines that put in data and look at outputs and that are doing constant training of you know, you, you have to. You say, okay, I take this in and I put it in and then I get this out. How similar is that to everything I've seen in the world?
Speaker 2:And I'll do that over and over and over again, because of the way that you design, build these things, you, you have to sort of it's like an A-B test, of like I put this in and then I get this out. Is that like humans do it? Okay, I'm going to try it. Six different, you know, a thousand different ways of these small tweaks, which is the closest to the way the human did this sentence? And you do that for every bit of knowledge, and so you only have so many knowledge, so much knowledge that you can test with, and the sum total of digitized human knowledge is the level at which they're testing, and so because they've reached that.
Speaker 2:They've reached that and then eventually what happens is, if you don't have a lot more here to test it against, then you sort of over-optimize the model to replicate exactly what people did in the past, right, you don't want that. And if a new thing happens, it doesn't know how to handle it.
Speaker 1:I get that and probably everybody gets that, yeah, but here's what I don't get. You know, there was a time going backwards now it was an expert system in which they were indeed putting in knowledge knowledge of how to play chess like a human plays chess, by putting that in. And then they did it with Go and they would get to a certain level not very far right, but they would get to a certain level. And then they changed it and they switched over to something like the large language model, something built on on uh operational transforms.
Speaker 2:That wasn't mine.
Speaker 1:All right, but anyway, they switched it over and they had the machine play itself. Yeah, right, so they started out with just the rules of the game, no knowledge of what humans have done with the game, no games by humans. They just had to play itself and it got better than any human way, better than any human at this point. Okay, so that's the scenario. That's the scenario in which it's arriving someplace that is other than where we've been.
Speaker 2:Yeah.
Speaker 1:Right, we people have been, and that's the situation. That's the thing that sits in my mind as the possible birth of AGI and more that kind of dynamic. It might not be that that's what a large language model can do, but they did it with Go. In other words, they did it with that other realm.
Speaker 2:Yeah, they did it with Go. But the thing is, with those games it is relatively easy to judge the results of the competition between the two sets of AIs that they're doing to do our improvements of. So you're able to have millions and millions and millions of rounds of games and you're able to judge which one was more like which AI did a better job of winning the game.
Speaker 1:And keep tweaking Right and keep tweaking.
Speaker 2:The difference is when we are judging it against human creation. We can't set two AI bots to just talk to each other and then have them determine which one was the better one. The only way to determine which was the better is to look at what people created in the past, and that's part of Well it does seem to be a lid.
Speaker 2:Yeah, we can get more optimized in doing it. We can get better at doing it. There's huge amounts of money and research going into all of these experiments, and so we may have a new breakthrough. But the current model of just providing all of this data in this large language model is in some ways constrained because you have to test against human creation and humans are only created so much.
Speaker 2:And so that's the constraint that people are doing and they're like well, maybe we can have ai do it, and then you get hallucination problems, or maybe you improve the quality of what it does, the ability to do the mining, all that kind of stuff, and that's the iterative improvements. We don't know what point someone will get a step change, improvement on this.
Speaker 1:Okay.
Speaker 2:Except that all of the AI researchers tend to believe that we will.
Speaker 1:Yeah, right, well, but they certainly have an interest in believing that, otherwise they could go nuts because they've made the wrong choice. But in any event, take, for example, the AI ability to map proteins, in other words to determine the structure of proteins, at a pace that dwarfs human pace I mean, it's not even in the same discussion and that arrives at correct model, at correct formulations of what the protein structure is. It isn't obvious to me that maybe that's more like becoming better and better and better at Go, but it wasn't obvious to me how some large language model approach was arriving at that kind of, indeed, creativity. Do you have any idea about that?
Speaker 2:I think the reason it works is because it's a more constrained search space. When I was in university studying AI, there was a definition of AI which was that AI is search. That basically the concept in the 1990s of AI and machine learning was all knowledge is out there, all possibilities are out there and the activity of AI is to go and find what thing you're looking for. It was entirely not creative. It was only about search, search and, in many ways, solving medical issues regarded to like proteins or genetics or things like that. They are search because there are only so many possibilities of chemical bonds to make.
Speaker 1:Oh, you mean search among possible answers.
Speaker 2:Yes.
Speaker 1:And then test the answer to see whether or not.
Speaker 2:So you can search among.
Speaker 1:That's not one of these language models. It isn't the network and the nodes. What do you call the whole thing? Neural network? Yeah, all right, so that doesn't sound like a neural network approach.
Speaker 2:But they are using neural network approaches, they're using large language models to test these things with like alpha fold and all these things like that. And you know I haven't read all the papers, but they're using all of the knowledge of scientific research and all of the you know they take. The way you do it is you take the really big models that you know GPT-4 and LAMA and Mistral and Clot all these really, really big models and then with all human knowledge, and then you train again on top of that with the specific knowledge of the field you care about and you say, okay, you know everything about the world and everything else and the way in which humans think. Now, only focus on all of the academic papers on biology, and you know cell mechanics and everything else. And then from there it's got to.
Speaker 2:You know it's like okay, look at this subset, look at this subset, then you trade it on the ways in which proteins work and then you have a testing function which says, okay, how do I tell whether or not you know? How do I simulate whether or not these proteins work together? And this is actually not entirely different than how normal software engineering testing works, like I've built web applications where, as part of the testing process, we just randomly went through and, like, changed variable values, and it would run through the own error program and change all the variable values over and over again and then you would see whether or not you could discover new unexpected errors in the application. And so what you're doing in this case is like I'm just going to recombine all these things and see if any of them within the simulator turn out to be viable and those ones then we can see would they in our simulation of this biological system have a positive effect? And then they can go test it in living systems.
Speaker 1:I want to clarify by asking you something that might be a misinterpretation. That's coming from what you're saying the large, you know, the huge models, gpt-4, et cetera. They're not a database. They're not or correct me when I've got this wrong they're not a gigantic database and then you ask a question and it searches the database and finds the answer to that. They're not that at all.
Speaker 2:No, no, it's compressed way down. It's a bunch of numbers. Yeah.
Speaker 1:Yeah, yeah at all. It's a bunch of numbers. Yeah, it's taken that, but it's turned it into really an incredible number of numbers at nodes. So it's nothing like a collection of information per se, it's something very different. And so all of a sudden it can do things that weren't programmed in. You know it can. It can all of a sudden have a capacity, what do they call it emergent.
Speaker 2:so there's an emergent capacity which nobody thought about putting into the damn thing, but which is just there and one, and one of the early stories is that when GPT-3 or whatever 2.5 came out, one of the engineers at Microsoft was trying to figure out how it worked and they asked it to create a unicorn. And this is a thing that only had text input. And GPT is like oh well, if I want to create a unicorn and I only have text, maybe what I'll do is generate a Python program that you could run that would generate a visual image of a unicorn and it was able to conceptually think about okay, you know, with what I have I can't visually create a uniform, but I do know how to program in Python and I know how to make Python output visual stuff, and so I can generate a Python program that you could then run that would make a picture of. You know, the original unicorn wasn't.
Speaker 1:You know these oval legs, but it kept getting better. I've seen that too, and that's your, that's your thing of testing it and making it better. But it couldn't have gotten better, am I right that what you're saying is the way it was tested was? They showed it to me and I said, oh, that's better than the last one. And then they do it again, and that's better than the last one, and eventually they've got a unicorn. And now the damn thing knows how to make a unicorn, and maybe it also knows how to make a horse and a dog and a cat and all sorts of other things is that right.
Speaker 2:But what they did is, once they realized that it could make pro like, once they realized that you know each new medium that it can create, then instead of just being like, well, does that code look right? They attached it to search engines to access the rest of the internet and they attached it to programming environments to be able to execute that code and look at the results. So they attached it to very different kinds of systems to tell, to do fit testing of did this do better than the last one? So you know, people are very slow with those tests and so the goal is to not have people do the like acceptance tests on these things. The goal is to build software they can do the acceptance test, because then they can do it in trillions of times and go over and over again learning it. And that's part of why these new large language models are so different than human intelligence, because that's not how biological brains learn. Brains learn and, like the, we are able to conceive of things and learn in our environment with really relatively little data input and create conceptual models, whereas the computers have just massive amounts of data input to try and do certain things. And you know one of the fascinating things is because it's all human knowledge and activity and everything else going into it, the results tend towards the median human who has attempted that task. So if you look at writing, it's the writing that these things produce, or the art that it produces is based on all of the people who are producing that kind of medium, and it gets about 50% in labor.
Speaker 2:When people who are using this to augment their work if they're really great at their job what happens is the AI makes them incrementally better. It makes them a little bit better, it makes them a little bit faster, it sort of automates some tasks, but it doesn't have a huge impact on their work. But if someone is below, like, and they're the bottom third, and they use this stuff, they'll be able to take their work and it brings them up to the median level of work, and so that has a huge implication for the labor markets around this stuff, because you can then generate very quickly. Anybody who is not talented could do the equivalent of this bar and it creates a floor in the level of the quality of the work, and so that's why we see, you know, scams and bots and all of the things sort of get to this level of good, and it's going to make it very hard to then determine what's a person, because they're not just like far off, not very good, but there'll be, like you know, the equivalent of the outer getting better.
Speaker 2:Yeah.
Speaker 1:And it could get better.
Speaker 2:I don't know that they'll actually get better than the median input of the source material like, unless we can train it to figure out who the best people are like it has to be another way it has to be able to learn how to judge quality in order to get better than median isn't't there another way, which is suppose it easily gets to the median?
Speaker 1:okay, yeah, now they pour in a gazillion dollars and they work for a long time and they get it so that it can go 5% better.
Speaker 2:Yep.
Speaker 1:Right, fine, now they go 5% better again, 5% better, again, 5% better. In other words, they take as the new, they become the new medium.
Speaker 2:Yep.
Speaker 1:And so the medium keeps climbing. I mean, I'm not saying this is going to happen, I'm just saying.
Speaker 2:I could you know I's part of what people mean by, you know AGI, artificial general intelligence is the point at which you can make these AI play chess against themselves, and when they can play chess against themselves, then they can get incrementally better and they will almost certainly go to places that a human intelligence wouldn't go to now.
Speaker 1:not necessarily, and that's already true, that's already true for the for chess and go into some stuff like that.
Speaker 2:So if it's true strategies that people wouldn't use, then it's likely choose true for for everything else.
Speaker 1:I was surprised that they're trying to do it with poker and they say they've succeeded. And since you can bluff in poker, that was disconcerting to me about how far along they are if they can do that, because it means the damn thing is sitting there and it has to have some sort of input of what the people sitting around the table. You know what I mean? Yeah, I don't. I haven't seen good good, you know reports on that? Um, okay, so we're talking. I'm not sure the average interest audience or the typical audience interested in ai for good reasons has other things on their mind. So I want to get to those things too, if we can.
Speaker 1:So, now we've situated AI a bit and we've gotten something of an understanding. You've got a lot, I've got a little. Everybody who's listening maybe has a little of how it's working and where it's at and where it might be going soon and what the impediment to it's getting sharper and sharper might be. But the kinds of issues that come up more broadly in society and in discussions is, for example and let me just do a few and then we can talk about all of it as you like Is AI an existential threat to sentient life and if so, how and what can be done?
Speaker 1:So that's an issue that comes up and that they bring up a lot as well. Or is AI not going to kill us all, which is what the first one sort of intimates, but perhaps, contrary to the rhetoric that abounds, is instead going to enfeeble and even infantilize us all? Or will it just be a wonderful aid to human development, solving problems and empowering, enriching and uplifting us all, with few of any negative, if any negative, consequences? And mainly, I guess, what will determine which way things go? So how about you? Start with the existential threat or not? Do you think that that has any real weight?
Speaker 2:You know, I don't know, no, but to me the fear of existential threat of AI feels like a Judeo-Christian apocalypse that God is going to come down from heaven and destroy the planet and all humanity and replace it with something.
Speaker 2:It's a millennial apocalypse myth that has been around in Western culture forever, and so we constantly think the new thing is going to cause this apocalypse to happen, constantly think the new thing is going to cause this apocalypse to happen. And for me, what I think about when I look at the AI that doomsday thing I'm like that's probably just our own fears about what's going on, and like deeply held cultural myths about it. And the only part of that that is probably real is that the AI is deeply trained on our own myths and knowledge. Like the reason the AI tried to convince the I think it was Kevin Roos, the journalist at the New York Times that he should leave his wife and fall in love with him is because the AI had read every story about malicious AIs trying to corrupt journalists and humans. And so the AI is like oh, I'm talking to a human trying to figure out if the AI is bad. I know what humans want when they are looking for an AI behaving poorly, so I'm going to behave poorly, just like every human myth about that's ever been written about poorly behaving AIs, so like it's a mimicking engine. And so the crazy part is, if there's one possibility that the AI goes bad and like starts killing humanity and like replacing us and everything else, the reason it would happen is because we taught it to do that, because we deeply embedded in our society and every discussion about AI and every discussion about science and science fiction, everything else, are like oh my God, the AI is going to kill us. And so the AI reads all the human knowledge and says, well, maybe I'm supposed to go do this.
Speaker 1:Okay, I think that's very clever, but I'm going to play devil's advocate, for I don't want to spend too long on this, because I sort of agree with you, but nonetheless. So now somebody responds. One of the critics of AI who knows about AI and who is saying that it's the end of humanity might reply okay, all that's true, all that is possibility. But what happens if ai's become as much better than us at all kinds of things as they are better than us at doing those games? Yeah, and ai's, and they have emergent properties, and among the emergent properties is some kind of a drive to engage with one another and interact and challenge one another, and blah, blah, blah. And they come to realize that we're annoying, that we're just little pests.
Speaker 1:This is what somebody would say. I mean, I've heard this right, that we are, in essence, annoying, annoying, uh factor on the planet and it would be a lot nicer if we just got rid of them. And so they get rid of us. That's what keeps people up at night. Doesn't keep me up, it does keep people up at night, and I wonder if there's an easy way to to beyond the fact that it would be our fault. Right, that's not going to relieve anybody of being worried about it. Okay, it's our fault. Is there any other reason why or any other mechanism that can prevent this kind of trajectory?
Speaker 2:I mean, I would say that the mechanism that prevents that kind of directory is we have a strong human intellectual tradition in environmentalism and, just like we as humans, like there are parts of the biological system that we have worked very hard to eliminate Smallpox, for example. We decided that we as humans don't like smallpox, this one virus, and we went in and we really really worked hard on eliminating smallpox. My suspicion is that if, in this magical superhuman AI future that it will consider us part of the ecological system that it exists in and that it created, and similarly to bacteria and animals and everything else, that, yes, we are destroying the rainforest, yes, we cause animals to go extinct, yes, we cause animals to go extinct, but we don't go out and, for the most part, attempt to cause animals to go extinct. They're inadvertent effects, is that if there became this all-powerful AI thing that it would perceive the humans as a part of the ecological system in which they exist and that probably would want to continue to exist that ecological system and, you know, would you know, maybe perceive it as an existential threat to it or not? I don't know, but you know, we're in this crazy land of science fiction and right now we don't know if we'll really get the number of fingers right, Like, so it's fun. So I guess my point is like it is fun to talk about that, but these language models and this AI are being used today and we can see where it's going to go, and it doesn't matter how scared we are of this doomsday scenario. But not enough people are going to believe in the doomsday scenario to actually implement a Japanese style. We're banning guns in our society and closing the ports to our country.
Speaker 2:There are examples in human history of looking at technologies and being like we are not going to do that. Examples in human history of looking at technologies and be like we are not going to do that. But our economic system and our political system today isn't going to say no to this stuff. Like there's too many incentives around pushing forward and adopting it. So what like? What we should look at is the reality of what we're creating today and the way it's being used, because you couldn't stop it.
Speaker 2:You can regulate it differently. China's regulating it very differently than they're regulating it in the United States. It's very different than how it's being regulated in Europe, than how it's being regulated in Europe, and you can change how it's being funded. How it gets funded in China is very different than how it gets funded in the West, in the US, and if you look at Mistral, which is the French giant French AI company, it's funded very differently than the American Silicon Valley companies. So you can set up different economic incentives, different intellectual communities, different ways of designing it, and right now we have three big ones we have the French model, we have the Chinese model and we have the Silicon Valley American model.
Speaker 2:The problem is because those are three fairly distinct ecosystems of how things work. If you wanted to stop it, you'd have to just stop it in three entirely different political and economic systems, and that's not going to happen, Because if you stop two of them, the third one would have massive benefit from the existing system. So I think that it is so much fun to talk about that science fiction thing, and so we keep doing it, and I think that distracts us from the very real impacts in the shorter term around work, around intellectual property, around creativity, around the future of productivity, and so it's fun, but it's, you know, one we can't stop. I find it funny.
Speaker 1:I find it funny more than fun, honestly, but there is something that does worry me honestly, but there is something that does worry me. So, supposing it's not going to kill us all, which I think is a reasonable supposition perhaps it's the opposite, or a kind of opposite impact, that it will enfeeble or infantilize us all. So what I have in mind and this is something I do worry about is that as AI becomes more competent let's call it and it can, for instance, plan somebody's week and it can write somebody's emails, and now we just fill in the blank with all the various things that it can do, and you look at the characteristics of all those various things and what's happening is it's doing more and more human things, the kinds of things that sort of define a human as a human, and the human is losing more and more of those things until, in a sense, we become the machines. In other words and I really think this is not so fanciful there was worry that when calculators were invented, people would stop being able to do math. You know basic math?
Speaker 1:Well, that's actually true. That is, it did happen. It's not the end of the world that it happened. Arguably, it's not even a big loss that it happened, but it did happen. And when people stop being able to marshal an argument, stop being able to generate evidence, stop being able to plan their own lives, stop being able to et cetera, et cetera, then I think it's a change in human behavior that is troubling. So what do you think about all that?
Speaker 2:I think that's a real concern, like we're continuing to hand more and more over to these things. They are not neutral in our interests. They are not neutral in our interests. They are very, very well-funded capitalist businesses that are about making money. These AI systems provide better trust and more like they're more reliable than what we can do on our own, than what our friends can do, what we learn in a school, and so at that point, you start trusting the AI more and you rely on it more. Now, will it stop us from being creative? I don't know.
Speaker 2:One thought I've had about it is that it will massively change the concepts of advertising, because if you start trusting these AIs to give you accurate information in all these different situations, then you're going to trust the AI to tell you what air fryer to buy and that you need to go buy a new electric car or all those things like that and so like.
Speaker 2:Then all of the entire world that we spend on advertising goes into just influencing the AI to drive you to make different kinds of decisions, and some of those decisions would be you know, make sure you go out and you exercise, or make sure you know, have you thought about taking the bus instead of driving or things like you know, go talk to your kids like socially positive stuff, and some of them will be by this brand versus that brand, or you need to buy more brands of things. So it's gonna. It's gonna increasingly shape what we're doing and how we see the world, and we're going to end up seeing weird things Like we know that humans are prone to believe in cults. Now what happens when someone creates an AI that's goal is to, like, replicate what L Ron Hubbard did with Scientology, or an AI that is QAnon and is pretty good and you can chat with it, but it's like it has these goals that are different things that it's trying to do.
Speaker 1:But these kinds of things, as with annihilation, are what happens when AI behaves in a nefarious fashion or people use it for a nefarious end. And the thing I'm worried about is different. It's what happens when AI is being used to try and make life better and the byproduct is that we're no longer living, and the byproduct is that we're no longer living. Let me give one example. So there's the example of the calculator. And you know it's so easy to solve every mathematical problem. I mean, imagine you had Mathematica in your lapel pocket, right, it's so easy to solve math problems that you forget how to do it. And for me, a GPS is like that. The GPS system is so easy and so accurate that I completely trust it and I use it to the exclusion of bothering to know where the hell I am or where I'm going.
Speaker 1:Okay, I saw there's this guy who does music commentary, mostly popular music commentary, a guy named Rick Beato. Do you know this guy? No, I, etc. And he sort of studied it and looked at the time when certain innovations were made, technical innovations in the creation of music music smoothing out voices, dealing with the beat of a drum and perfecting that and so on, all technically. And he said look, what seems to be the case is that, as it gets easier and easier to generate music, there's no reason and it seems like a waste to become personally good at that, which is analogous to. It's a waste of time to learn how to add, you know personally, as compared to the, or to learn where everything is and how to get to it.
Speaker 1:And he's basically saying that same phenomenon is already happening regarding music, and so there's a dearth of effort by humans to become better at creating music and to create better music, because there's no point, because you know it's so mechanical and it's so, and that's what I'm, what I'm sort of worried about, especially when you start thinking about it, as it's easier to plan your week by relying on this thing that you have in your pocket and not thinking about it, or it's easier and now they're, you know, pretty soon it'll be it's easier to have an emotional conversation, as you gave in your example, without with this thing, which is more understanding, seems to understand me better than anybody does. Blah, blah, blah, sure.
Speaker 2:It follows me. Those personal AI bots are super popular. The way I think about it is before the advent of photography, there were many professional portrait painters, and they were not making portraits of everybody. They were making portraits of people who could afford to pay a professional portrait painter, and those people's goals were at the time, to very accurately and positively portray what you would see, and you couldn't make a way of representing what someone actually looked like without painting it. And then, once photography came along and then we were able to create a portrait with photos of someone, then the field of painting was not constrained by accurately representing reality and you've got this massive wave of creativity in painting.
Speaker 1:This is the optimistic formulation. Yeah.
Speaker 2:And so what? Like? Yes, there was on a bunch of this stuff, the ability to do things before where you had to put a lot of time, you had to spend a lifetime of practice, and so now we see massive amounts of stuff being done by people who don't make quality stuff. The question then becomes can we set up the system to discover the people who then use all of those tools to do something too creative and neat, and does it feel like none of that is happening because the amount of content being produced has gone up exponentially? So my theory is, the level of people doing the creative work is roughly the same. It's just now buried under a mountain of other stuff, so it feels like no one is doing the creative work anymore. So because I think that, using MusicML and all these new music creative stuff, yes, most people who use the AI music stuff make covers, but you know what stuff make covers, but you know what Most working musicians probably are cover bands.
Speaker 2:Like they're playing music, live music at bars and you know weddings, and like you know neighborhood festivals and things like that. They're not, and that is actually the way local musicians were doing 200 years ago. Like they may be playing hits. They may be playing stuff they can. There were no star performers. There were star composers, but no star performers, and so those folks are still doing that. We just now have people to be able to be the stars and you know, it feels like because everybody gets the tools to be pretty damn good at it it feels like we've got a bunch of mediocrity. And that's that same thing the way that writing works. It takes the lower half and brings it up. It gives you auto it up, it gives you auto tune, it gives you, you know, beat tracks that make sense. It gives you transitions for stuff. And then the question is like, what do you do with it? And it doesn't.
Speaker 1:it can't replace the best yeah, but I think see that I'm not questioning anything that you just said I I think it all makes good sense, but I don't think it's addressing what I'm worried about.
Speaker 2:Yeah.
Speaker 1:I'm not worried about, you know, less of the top I'm worried about. Or the same amount of the top but not more of the top, or anything like that. I'm worried about the AI not killing us, right, but steadily taking over, not because it's maliciously taking over, but because it's just a process, but taking over much of what humans do that makes humans humans or that makes us fulfilled humans. That's a different concern, right, fulfilled humans? That's a different concern, right?
Speaker 1:So I was using the example of the music thing, which does have the effect that you were responding to, merely to say look at the dynamic of the, of the person who's into music, no longer being into it in anything like the same way. Yeah, right, being just not feeling the desire to do that. For your example, what happens when the robots are playing the instruments? Do you then learn how to play an instrument? The same kind of dynamic arose with, say, chess or Go, where people were saying well, will anybody keep playing and go through the effort to become good when this other thing is so much better? And it's an open question, I think, um, people still play go and they still play chess yes, but the guy who got beaten in the crucial evidentiary episode gave up right after.
Speaker 1:And you know, again, it's not at the top, it's not the professionals. Later on it will be because the young person might not go up. I don't know whether this is happening. I'm just saying it is something that I actually sort of worry about, as compared to worrying about a robot army sweeping over us.
Speaker 2:There's some definitely points there and there's some validity to it and there will be things that we stop doing as much. But you know we stopped washing our clothes by hand and used washing machines.
Speaker 1:You know we, that's not the same as stopping to plan your own life, you know, relying entirely on this stupid little box that talks to you to decide what you're going to do this week. That's sort of different, I think.
Speaker 2:I think it is and I think we will freak out and find it crazy. But you know, there's different generations, like my kids are teenagers and their preferred source of like entertainment is watching live streams of other people play video games, and they could play the video games themselves and they do play the video games. Play the video games, but they actually they. They don't watch very much produced stuff from Netflix or things like that. They don't watch even other you know, narrative stuff. I think the entire world of what they watch as the primary thing is they play video games and then they watch other people play video games. Sometimes they watch other people play video games, sometimes they do both. They have one screen where they're watching and listening to someone else play a video game while they're playing a video game maybe the same game.
Speaker 1:And to me that's totally crazy and you're not concerned, right. But it's not just crazy You're not concerned that there's an element of addiction.
Speaker 2:I mean, yes, and one of the tricky parts is not just in video games but all of what we're creating with this stuff. It's much better at triggering the psychological elements of addiction and the people designing these systems you know, there's like all of the tech folks working on this stuff have read the pop psychology books and they've read the papers and they are like intentionally building addictive systems because that's what gets rewarded in capitalism yeah when I was in college yeah a long time ago.
Speaker 1:um, we discovered because in the community where we were living there were kids, and they were. So it's a long, I'll make the long story short. They were, um, sniffing glue which is totally, you know, debilitating. Ultimately it just rots away brain cells and they were doing that. And then we looked into it and we realized that the ingredient in the glue that people were turning to because it, you know, gave them a kind of high, had nothing to do with the glue being glue, to do with the glue being glue. It was put in there so that these kids would buy lots of glue to sniff. It's not so different from oxycodone and all the rest of it.
Speaker 1:Right, it was the first time I encountered anything like that, you know, and it was nauseating too. We have covered the few questions that I sort of assembled for the purpose, but that's me assembling. What about you assembling? Is there something else that you want to talk about that I've left out?
Speaker 2:I mean, the thing that I want to have us talk about and think about, when we have AI and specifically thinking about the Revolution Z, the audience and your work is who controls this stuff? How is it being applied, and can we make it a tool for sort of a more humane expression and creating a fairer economy and collaborating better? You know one, there's a science fiction book I read called the Half-Built Garden, and it's a solar, punk, positive science fiction book and in it they have this idea of this AI-driven computer network called the Dandelion Network that essentially does a really good job of, within a community of people, understanding people's different views and helping people come together for collective decisions. And in this case, the AI is clearly controlled by the people who are using it, and they're using it as a tool to make sense of themselves and to come to collective decisions. And so in this sort of fantasy future of AI, people engage in all sorts of discussions and they come together and everything else, and then the AI is able to say hi, this is what we've. You know, this is what this community seems to be thinking about, and here are the options, and this is why people want to do these things, and so I think we can design AI systems very clearly that do that. I think we can design AI systems that change the nature of work so that we get to do more of the socially interesting work and less of the work we don't want to do. And so what one option would be is well, let's have only 1% of society get to do interesting work and everybody else is unemployed and doesn't get to do any interesting work.
Speaker 2:And the technologist folks in Silicon Valley who look at it, they're saying, well, we need universal basic income because we're going to have these massively profitable corporations that have almost nobody working for them, that run almost all of the economy, except, you know, all of the intellectual parts of the economy. And so you will have a certain level of physical work that needs to happen like complex physical work that robots might not be able to figure out how to do for a long time. And then you have a small class of super elite intellectuals who control the AI systems, and then you have everybody else in the middle and there's no point to them. Like, what is the point of a teacher when you could have one person record all of the lectures and talks and then you could have an AI thing that sits there and works with each student individually and helps them understand what's going on, like what's the point of any kind of intellectual work, any kind of office work, if you could have an AI system replace it comes.
Speaker 2:How do we create economic institutions and how do we create businesses and co-ops and other things like that in a way that uses this stuff to empower the kind of work relationships we want and the kind of economic relationships we want, instead of just a few super wealthy corporations that control everything, employ almost no one and manual labor. And the current option, oddly enough, is adopting ideas from Martin Luther King when he was doing his Poor People's March, which is this universal basic income, which is like we can't think out what you would do or why you would have an active role in the economic institutions of your life, and we don't think you should have any political or managerial say in how that works or what you do, so we'll just give you a certain amount of money every month and, because you're superfluous to the economy, that's going to lead to a lot of alienation.
Speaker 1:We want to be a part of things yeah, I, I mean, I agree with you, it, it echoes, but at a completely higher level of, I think, urgency because of all the issues involved the beginning of social media when similarly, um, you know, you could see, there are these intrinsic features of it, the way it's developed, that are going to be harmful to people's attention spans, to all sorts of things. But it could be better. And how do you figure out? Well, it was true, it could have been and it could still be, but it could have been much better. That's the kind of thing you're working on. And so the same thing is true, I agree with you.
Speaker 1:I agree that AI could be used in positive ways, purely in positive ways. That would have, you know, dramatic positive effects on human well-being and capacity and so on, absolutely, and I even think we know how the institutions could be constructed. Now, this might be egomania, but I do sort of think, you know, participatory economics would allow for all of that, permit all of that, prevent all the negative incentives, et cetera, et cetera. So I do think that, but that's nice, except there is this problem of getting there and of keeping this stuff from becoming worse and worse in its impact, as did social media and about that I don't, you know, I'm not at all sure.
Speaker 1:I think that the it's going to be interesting to see what happens in September and October regarding the US election. You know the level of actually false information and false videos and false audio and false everything that's going to fly all over the place, everything that's going to fly all over the place. It may make a shambles of having you know, of making believe that an election is actually something other than a total manipulation. If that happens already because of AI I mean, there's already a lot of it, even before AI but it's, you know, an exponentially greater possibility that lurks not far away.
Speaker 2:And, interestingly enough, we've now made the decision that we are not going to control essentially not going to try and control misinformation on social media platforms Like that. Four years ago, even two years ago, there were a commitment from the people running these platforms that they would try and stop coordinated, inauthentic activity. So that's propaganda, whether or not it's a non-state or a state actor, things like that, and we now know both that that is much easier to do than it was several years ago, and the political environment and the economic environment, the pressures around it, have caused the platforms to walk away from the idea of doing something about it. So when there was the assassination attempt on Trump, there was almost no information taken down. Like there was not a massive effort on the part of the platforms to control rumors and drive people to mainstream media outlets. They were just like we throw up our hands everybody posts everything.
Speaker 2:And so it's going to get worse in that way, both because of the platforms and because you can now create. You can now create.
Speaker 1:Produce it.
Speaker 2:You can produce it much like. The cost of production is going way down and we now see that with the collapse of search engines driving traffic to webpages, because you now no longer trust any webpage because they all can be sort of very easily artificially created. You know I use this tool Reload. It's like AI created thing and I can create an incredibly professional looking entire website In five minutes In a minute and a half.
Speaker 1:Right, I was going to say in a minute and a half.
Speaker 2:And like it's up on a domain name and it looks like something that would have taken weeks or months to create, and so you basically can sort of flood the field of all of these things and no one knows and you can no longer know what's going on on any of them. And my worry is that when you get moments of you can't trust anyone and no one knows, then that's the moment by which people say we must go to a strong man, we must go to this kind of authoritarian leader who can just slice through it all and tell us the truth and may be wrong, but at least they're consistent and they're dealing with it.
Speaker 1:And I don't have to feel so amorphous and so pulled in every direction. I can just go one way.
Speaker 2:Yeah, and it all lies and it's all inconsistent, and I have no idea what any of it means, and so, therefore, I'm just going to pick someone who is doing it and then I'm going to who's? Entertaining.
Speaker 1:Yeah, and who's entertaining?
Speaker 2:Yeah.
Speaker 1:And Trump, but in any case, something parallel to what you're describing about social media I think happened with AI, because when AI was first getting I don't mean 40 years ago, I mean like three years ago or whatever, maybe it was four years ago they were all putting out these advisories and I don't remember them all. But, for example, do not connect AIs to the internet, do not let AIs prompt each other, do not, et cetera, and they were perfectly sensible there were lots of sensible ones all gone. They aren't even gone, they're obliterated.
Speaker 2:The entire purpose of being of OpenAI was to create a nonprofit that had accountable external controls so that they would do it responsibly. And the economic incentives of this stuff got to the point where they took an organization that was entirely created to handle these economic and social incentives and it became the reverse yeah.
Speaker 1:That's remarkable. I mean it's predictable, but when you see it in that it's like you know. I know that cancer hurts, but if I get it it's a little different than the abstract knowledge. And that's true for this too. I mean I would have said all this was going to happen. I did say you know, but there's a difference when you sort of see it unfold so dramatically and so pervasively. I don't want to make the audience depressed, but it is a difficult situation, it certainly is.
Speaker 2:Yes, it's a difficult situation and there are strong arguments for different things. There's a strong argument that it shouldn't be open source because everybody who has it gives the keys to the nuclear weapons to everyone.
Speaker 2:On the other hand, if we don't open source it, the way Mistral and actually Meta is doing lots of open source work on this stuff if we don't open source it, then it ends up in the control of a few very, very wealthy corporations. And so you know. The irony is that China is doing a really good job of regulating the really good AI inside China because, like they have academics who are checking with people, their companies need approval of the Chinese government for what kind of stuff they're going to roll out, so they understand the implications of it. Now they're doing it to reinforce the authoritarian Chinese state, but they're actually creating a real regulatory framework for it, whereas in the West we have two options. We have this open source free for all thing where, like, let's just give the weapons and the tools to everyone, or we have this a couple guys get to decide everything within. You know, microsoft and Google.
Speaker 1:And actually you have a combination of the two, that being Trump, who will eliminate all regulations and simultaneously exert authoritarian control. So it's like the best of every world. I mean the worst of every world, I mean. The odd thing is I'm trying to end on a better note the odd thing is we have a tendency to see the disaster scenarios, the painful scenarios, the hurtful scenarios, scenarios a lot clearer than we see the positive scenarios. And so, even while Trump is rising you know, sanders is the most popular politician in the country, but we're all you know, we can't grok that. Second point it's like that can't possibly be the case. What's going on?
Speaker 1:But it is true that right now, there is, in some sense, more progressive thought, more even radical thought, afloat in the US not in institutional power, right, but afloat in the US than there has been. And the problem is and we can't lay this on them the problem is that we haven't done a good enough job, we being those who are trying right. I haven't done a good enough job of cohering all that and acting on it. I mean the, the thing that makes me sort of saddest in some sense.
Speaker 1:If you look at project 2025 and you look at, you know the trump If you look at Project 2025, and you look at, you know the Trump thing and you look at their plans for replacing I think it's 50 to 100,000 government employees with Trump selected. You know MAGA people. They're playing chess. They're playing hardball, but they're playing chess, you know. In other words, they're playing at a very high level. We are maybe playing checkers or tiddlywinks, and yet we're still in the game and that's because we're right. In other words, justice is better and compassion is better and so on, but we're not very good at fighting for it, or so it seems to me anyway.
Speaker 2:We are very good at making the argument and convincing people of views, of worldviews and culture. For the vast majority of Americans in the US case, for the vast majority of Americans in the US case, we are winning the intellectual, cultural, ideological fight in ways that are like if you just look at the acceptance of trans people like it is really fast and it is really effective and it is really profound and it's exactly right.
Speaker 2:We are losing the institutional fight to a group of people that don't even care about winning the hearts and minds and ideas and beliefs of the majority of the people. They are perfectly happy to take institutional control and power with a teeny minority and have given up on convincing most people.
Speaker 1:But they are preparing a force of people to fill slots and they are developing clear program, institutional program. You know, not just. I think you're absolutely right. I think this has been going on since the sixties. I mean, I don't know about before, but it certainly started with us winning the cultural battle, winning the ethical value battle, winning all sorts of things and ignoring the need to solidify the victories. It's like taking ground in a battle to solidify the victories with institutional structure and with you, you know, and it's been happening ever since.
Speaker 2:And the same thing happened with the anti-globalization movement.
Speaker 2:Like one of the big things that came out of the anti-globalization movement, I mean, we stopped a bunch of big treaties. But another thing was in the media and a media activism movement that was very participatory, it was proto-social media and we had the voice you know be the media, a media revolution to make revolution possible, all of these things and the idea that people and activists and everyone should have a voice and be able to create their media. That did get adopted. But the other half of it, which was we should own the means of communications, didn't happen. And so we got the structural stuff of everybody now gets a voice and to get able to participate and you don't have to have a printing press or a radio transmitter we got that part. You don't have to have a TV press or a radio transmitter, we got that part. You don't have to have a TV channel, everybody got this voice. You were streaming video, all these things, this podcast, for example. We democratized that part, but the ownership of the infrastructure ended up with a new set of very large corporations.
Speaker 2:And so it's very similar, like we changed the way in which people participate in creating media massively, fundamentally, but we just created a new set of owners of the means of communication.
Speaker 1:And who still are in control. Yeah, there was another thing that reiterates some stuff that you said in the earlier episode that we did. People might want to look that up. There was another thing that you said that I was struck by in that earlier session Because I was asking you at the time about your efforts to create social media and working on doing something now, yep, and you said that there was.
Speaker 1:You could sort of view it as two aspects. One aspect was what's under your control. One aspect was decisions you can make and choices you can implement and ways you can behave. But the other aspect was what the surrounding population, culture, constituencies would relate to and would use, hopefully in a positive way. And if you didn't have the second part, the first part was sort of doomed. You know there was only so much you could do because you needed that second part, and I think that's right. And you know I've encountered that all my existence and it's still the case and at some point it will hopefully turn around. I think it will progress, not only in ideas and values, which can be rolled back, but in ideas and values and structures and institutions. And now it can't be rolled back and hopefully we'll still see that happen.
Speaker 2:Yeah, I mean I hope we will, and we need to keep fighting and we make progress.
Speaker 2:And we need to keep fighting and we make progress and we try and learn from the past, and we don't know where it's going to go.
Speaker 2:But the new AI stuff and the new tech does give us an opportunity to set a new set of rules.
Speaker 2:It does give us an opportunity to run these experiments again, and every time there is a major medium shift in the technology to the printing press or to radio or to television or to, you know, copier machines to make zines all of these transitions usually replace the institutions that primarily operate on them, and so we have the opportunity especially because at least some part of this new AI stuff is open source and we have this tremendously huge open source technology commons that people have contributed to.
Speaker 2:We have the opportunity to build a new set of kinds of institutions and a new sets of way of working that use the resources of capital to build these things but run it autonomously and run it independently with different institutions, and that's one of know, that's one of the exciting parts about the fact that there are, you know, some of the big corporations in this space who are investing hundreds of billions of dollars, have, for their own interest, decided that they need to be open, they need to release it to everybody, and that gives us the opportunity to not just be users of chat GPT, but to be able to build alternative systems and things that we can run and control, and so that's one of the exciting parts is, at least we have a third model which is open, that isn't just the Chinese or the Silicon Valley controlled corporation.
Speaker 1:I don't think we have time to discuss this now, but a technical advance that I think is probably not far off, I think may have mind-boggling implications. And it's a portable perfect lie detector, that is, imagine that your phone sitting in your pocket would tweak you every time it heard a lie. I'm not even sure what the results of that would be, but I think it would be very, very big.
Speaker 2:It's not going to be 100% accurate, but we do know that there are lots of tells around honesty and equality, and we could also like there's also the ability to check things against an infinitely huge bit of, you know, body of knowledge.
Speaker 1:Yeah, but I mean really, your partner can't lie to you, yeah, your kids can't lie to you. Partner can't lie to you, your kids can't lie to you. You can't lie to them. You can't lie at work, you can't lie anyplace, because it's immediately known to be a lie. Now, maybe it's impossible, but it does strike me as looking like something that could emerge pretty soon. Something that could emerge pretty soon.
Speaker 2:I think it'd be possible to detect something that created something that and I think this is actually pretty relatively far off that could detect when someone knew they were lying.
Speaker 1:Oh, okay, that's true.
Speaker 2:But if someone really believes what they're saying and it is a lie, then you don't have those tells. You don't have Because there are things that we do the way we. If you really believe it and it's a lie, then there's nothing you can do.
Speaker 1:And that's often the case, of course. We're now an hour and a half. I'll ask again you got something more that you want us to talk about?
Speaker 2:No, I think we covered the issues of what's going on in AI and the things that are going on in it. What I would say is that when I first realized the implications of this, I felt like I was going through my own situation, similar to the scientists on the Manhattan Project, where, to the outside world, nothing has changed.
Speaker 2:But, you know that something has been created that will fundamentally potentially tear apart everything that's going on, and when technology gets adopted, it is both faster and slower than we think and inconsistent.
Speaker 2:The future is here, it's just not evenly distributed. So what I would say as a result is we need to be paying attention to what's going on, because things will happen in small areas and small things that are happening and small experiments, and then very quickly get pushed through all sorts of institutions, and so we can see and shape and understand it before it gets pushed through and have a willy to react to it. If we're paying attention to those experiments that are going on here in the small things, but by the point at which it hits a threshold and gets massive adoption, that's like a dam breaking. All you do is cling to your raft. And so I think that what we need to do is realize that there's going to be wave after wave of massive changes to our economic, social, political institutions driven by this AI, and we don't understand the way exactly it's going to work and how it's going to play out. But just because you don't see it tomorrow and because it feels like a cute little chat bot doesn't mean that it isn't going to happen.
Speaker 1:Yeah, I agree with you. The only thing in that section that you just said that struck me as wrong is that the people in the Manhattan Project were remotely like you, Because, sadly, everything I've been able to understand about it and it is, I mean, you know, as you described it it's a sort of a fascinating thing that happened. Setting aside the magnitude of the crime, they weren't doing that, that there was virtually no discussion of the crime. They weren't doing that, that there was virtually no discussion of the social, political or economic implications of anything. They were instead, I suppose you could say, technocrat. They were taught at places like where I went to school MIT, and they just had imbibed that you don't ask those questions, you know, you don't talk about that stuff. You just solve the problem. You just solve the technical problem and that's what apparently happened.
Speaker 1:And I got that from a number of people, including the person who armed the bomb that was dropped on Hiroshima and Nagasaki. In other words, at the last minute you have to. You know, like in the movies, you have to send it to be alive. That person said the kind of thing I just said about, and he became a leftist. That's how I was able to know him and talk to him. I think it's a little better now than it was then. There are more people like you in the world of AI. Maybe what's his name at OpenAI was that way and transformed.
Speaker 2:No, Sam Altman was always a pretty unethical mess.
Speaker 2:Okay so then forget that. What I would say is there is, amongst AI researchers, a kind of Christian evangelical mysticism in their work. That is very weird and very different than things that we have seen before and very different than things that we have seen before that there is a strong tendency among the people who are building the AI systems to see themselves as bringing about the second coming of Christ. Seriously, yes, they see themselves as bringing about. They fear they're bringing about the apocalypse, but they dream that they are bringing about the movement of humanity into heaven.
Speaker 1:Are you describing this as an analogy or that they actually are thinking in terms of God and heaven and so on?
Speaker 2:No, no, not analogy.
Speaker 1:They're worried about bad and they're seeking good. You're not saying that. You're saying they literally.
Speaker 2:They believe that these things can be potentially, at some point in the future, an all-knowing, benevolent intellectual force that humans have birthed into existence that will benevolently take care of us and solve all of our economic and social problems. They will create a god, that is, the computers. That will then be an active participant in our world, and there's a fear that it will be a malevolent god or a benevolent god. But there's actually an amazingly weird, very weird intellectual, social like religious tradition that exists within the AI researchers that we don't talk about enough because that's very different than the MIT engineer of the past Now, a lot of them went to MIT but it's very different than the way like there is a religiosity, there's a religious belief part and the fact that they are on a religious crusade to what they're doing.
Speaker 1:They need some sort of rationale, some sort of way of justifying what they see as, and what may turn out to be, their incredible power and influence, and so they come up with this as a rationale. I'm not sure it's clear what the difference is, but the difference is sort of a deep seated religious belief that they were little kids that you know they have this religious commitment, versus they're constructing a rationalization for their behavior.
Speaker 2:I mean, I don't think most of these folks grew up religiously Like. Most of these folks are, you know, a bit on the autistic spectrum. They're scientists, engineers, physicists, mathematicians, like the same generation, same kinds of people who created the transistor and created the internet and created all of the technology innovations of the last 50 years. But today, when they look at what they're doing, they're adopting a much more religious perspective on it and understanding of it, and a particularly techno-evangelicalism that feels like a neo-Christian evangelical movement around what they're doing.
Speaker 1:At risk of going on too much longer, I do want to ask you one question about this Do you think, about yourself personally, how to address that, to impact it? In other words, this is your field, this is where you know people, this is where you I'm wondering if you and I'm not being critical I'm really sort of wondering are you reduced in some sense, to just watching it and having it be there and that's the way it is, or do you think in terms of impacting it and changing it?
Speaker 2:I mean, so I don't know that I have the like.
Speaker 2:There's only so much work that each person can do and how they can do it.
Speaker 2:And so there's the work of Aza Raskin and Tristan Harris, who have the Center for Humane Technology, and they've done a lot of work on this stuff, including a lot of speaking on it in really good ways of trying to reach out to the world and trying to approach these folks, and there are people within these organizations that are doing it, and there is a massive internal fight within these organizations. The reason that the company Anthropic exists is because they were engineers at OpenAI and scientists at OpenAI who didn't feel that OpenAI was being ethical in the way it was working and they wanted a different structure and a different set of meaning behind it. And so at the moment, within these communities, there is a lot of debate going on, but the trick is, in order to be able to participate in the debate, you have to be at a certain level to be able to also be creating the technology. Otherwise your views don't count, and so I haven't dedicated my life to that kind of work, and so.
Speaker 2:I've been working on social media protocols, which is my specialty, and I'm aware of the AI stuff. We use the AI stuff, we follow it, but there's only so much each person can do.
Speaker 1:I get it, yeah, I get it All right. Well, I guess maybe we should, rather than open a whole nother topic and continue on, bring this to a close. Thank you for doing this. I know it isn't your literal area of concern, but still in all, it's become quite obvious that you are very thoughtful about it and have had things to say which I think the audience will find fascinating. We'll see. And that said, this is Mike Albert signing off until next time for Revolution Z.